Generative AI, also known as Generative Adversarial Network (GAN), is a cutting-edge technology that enables machines to generate new and original content, such as images, text, or music, that resembles human-created content and displays human intelligence. This article will provide an in-depth understanding of generative AI, its workings, applications, benefits, limitations, ethical considerations, and future potential.
It is also important to note that generative AI relies heavily on computer code and algorithms to function effectively.
Understanding the basics is crucial, so let’s start with an introduction to generative AI. We’ll then explore how generative AI works, including the underlying concepts of machine learning and generative modeling. We’ll delve into the techniques used in generative AI.
Generative AI has a wide range of applications across various industries. We’ll explore its use in image generation, text generation, music generation, and video generation. By examining these applications, we can understand how generative AI is revolutionizing creative processes.
While there are immense benefits to generative AI, it is essential to be aware of its limitations. We’ll explore the advantages it offers, such as creativity augmentation and enhanced productivity, as well as the limitations, including the difficulty in controlling the output quality and the need for large datasets.
Ethical considerations play a significant role in the development and deployment of generative AI. We’ll examine the ethical implications, focusing on fairness, bias, and the potential for misuse and manipulation. It is crucial to assess and address these concerns to ensure responsible use of this technology.
Looking ahead, we’ll discuss the future of generative AI. Advancements in technology are expected to further improve the capabilities of generative AI systems. We’ll also explore potential applications that could disrupt industries and shape the future.
Key takeaway:
- Generative AI maximizes creativity: By using generative modeling techniques, such as machine learning, generative AI enables the creation of new and original content in various domains like images, texts, and music.
- Generative AI has versatile applications: Generative AI can be used to generate realistic images, natural language text, and even compose original music, opening up new possibilities in fields like art, entertainment, and advertising.
- Ethics play a crucial role in generative AI: Fairness and bias need to be considered when developing generative AI models to ensure that the outcomes are unbiased and inclusive. The potential for misuse and manipulation highlights the importance of ethical guidelines and regulations in this field.
How Does Generative AI Work?
Generative AI, also known as generative artificial intelligence, utilizes algorithms and machine learning techniques to produce original content. Now you might wonder, “How does generative AI work?“
Let’s discuss the process step by step:
1. Data collection: Generative AI algorithms require a substantial amount of data to learn and generate new content. This dataset can consist of various types, such as text, images, or audio files.
2. Training: The collected dataset is used to train the generative AI algorithms. During this stage, the algorithms carefully analyze and process the data to construct a model. They learn the underlying patterns, styles, and structures present in the dataset.
3. Generation: Once the algorithms are trained, they possess the capability to generate new content based on the learned patterns and styles. This includes the ability to produce realistic images, generate coherent text, compose music, and even engage in human-like conversations.
4. Iteration: Continuous refinement and improvement are achieved through multiple iterations of the generative AI algorithms. By incorporating feedback and adjusting parameters, the algorithms become increasingly accurate and sophisticated over time.
5. Applications: Generative AI finds applications in various fields such as art, design, music, gaming, and healthcare. It assists in creative tasks, offers personalized recommendations, and aids in research and development.
Here’s a useful tip: When utilizing generative AI, it is important to consider ethical implications and potential biases present in the data. Regular monitoring and evaluation ensure that the generated content aligns with desired outcomes and values.
So, now you have a better understanding of how generative AI works!
What Is Machine Learning?
Machine learning is a field of artificial intelligence that focuses on developing algorithms and models that enable computers to learn and make predictions or decisions without being explicitly programmed.
It involves using mathematical and statistical techniques to analyze and interpret data, allowing the computer to improve its performance over time. One important aspect of machine learning is its ability to identify patterns and relationships in data.
By training a computer with a large number of labeled examples, it can learn to recognize and generalize from those patterns. This enables the computer to make accurate predictions or decisions based on new, unseen data.
Machine learning is used in various applications, such as image recognition, natural language processing, and predictive modeling. In image recognition, algorithms are trained to identify and classify objects or features in images. In natural language processing, machine learning is used to analyze and understand human language, enabling applications like voice assistants and language translation. Predictive modeling uses historical data to make predictions about future events or outcomes using the internet.
Machine learning has revolutionized many industries, from healthcare to finance to e-commerce. It has the potential to enhance efficiency, accuracy, and productivity in various domains. It is important to consider the ethical implications of machine learning, such as fairness and bias, as well as the potential for misuse and manipulation of the technology.
What Is Generative Modeling?
Generative modeling, also known as generative AI, is a fascinating field within artificial intelligence that revolves around the creation of new data or content based on existing patterns and examples. This technique is achieved through various methods, including autoencoders, variational autoencoders (VAEs), and generative adversarial networks (GANs).
Autoencoders serve the purpose of learning compressed representations of input data and, in turn, generating fresh data by decoding these representations. On the other hand, VAEs learn a probability distribution of input data, allowing them to generate new samples by sampling from this learned distribution. GANs, on the other hand, consist of two competing networks: a generator network and a discriminator network. While the generator network creates realistic data, the discriminator network distinguishes between real and generated data.
Each of these techniques possesses its own strengths and limitations. Each of these techniques possesses its own strengths and limitations. Autoencoders and VAEs excel at generating similar data to the training data, making them highly useful for applications in image and text generation.
GANs, however, are renowned for their ability to produce highly realistic content and have been widely utilized for tasks like generating lifelike images and deep-fake videos.
The impact of generative modeling extends far beyond the realm of AI. This innovative approach has opened doors to new possibilities in fields like art, design, and entertainment.
By allowing the creation of unique and captivating content, generative modeling inspires and engages audiences. That being said, ethical considerations must be addressed, particularly concerning the potential misuse and manipulation of generated content.
This progress will undoubtedly create opportunities for the application of generative modeling in areas like healthcare, robotics, and virtual reality. As the field evolves, the potential of generative modeling to revolutionize digital content creation and interaction becomes increasingly evident.
What Techniques Are Used in Generative AI?
Generative AI utilizes various techniques, such as autoencoders, variational autoencoders (VAEs), generative adversarial networks (GANs), and recurrent neural networks (RNNs), to generate new data based on learned patterns.
Autoencoders are neural networks that learn and recreate input data, enabling the generation of new data. VAEs are a variation of autoencoders that not only recreate input data but also encode it into a latent space, which can be manipulated to generate new, similar data. The encoder in VAEs plays a crucial role in converting input data into a smaller, more dense representation, while the decoder is responsible for reconstructing the original input data.
GANs, consisting of a generator and a discriminator network, generate new data while improving its quality through competition. RNNs are used to generate sequential or temporal data, such as text or music.
A notable fact about generative AI techniques is that VAEs have successfully generated realistic images, such as faces and landscapes. These models can produce new, diverse images that resemble the style and features of the training data.
Applications of Generative AI
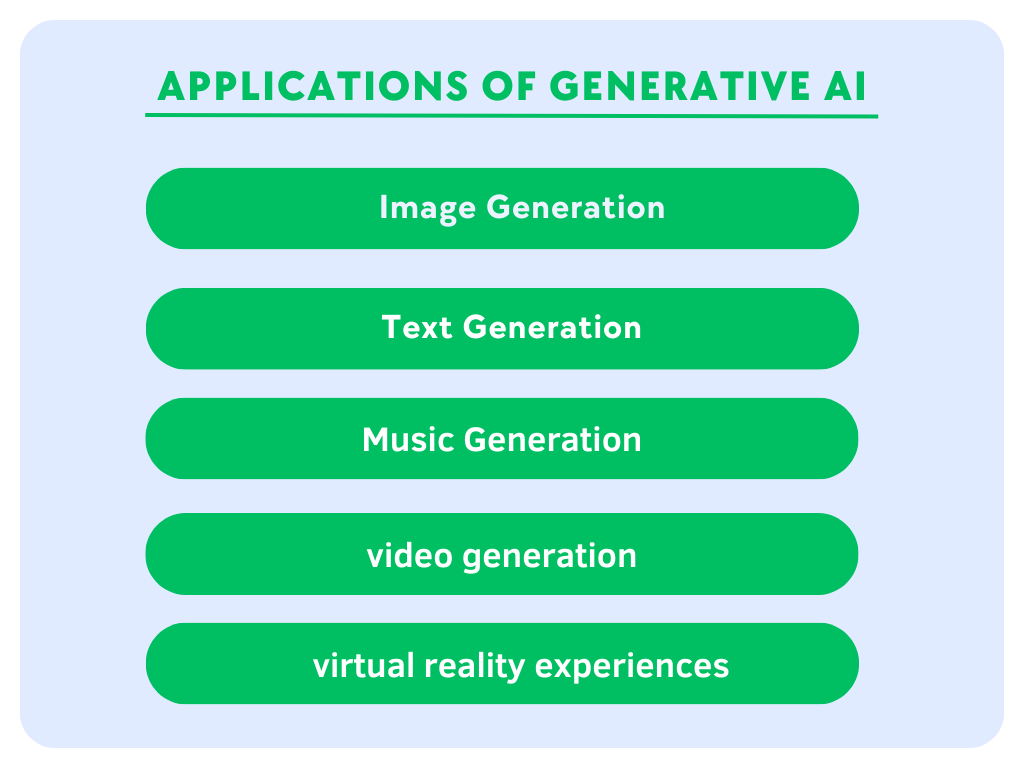
Discover the endless possibilities of generative AI through its diverse applications. Unleashing the power of generative models, we will explore the realms of image generation, text generation, and music generation.
From stunning visual artwork to captivating narratives and enchanting melodies, prepare to be amazed by the creative outputs that emerge from the realm of generative AI, including the use of large language models (LLMs) for tasks such as essay generation, code development, translation, and understanding genetic sequences using various modalities.
Image Generation
Generative AI creates realistic or artistic images. It generates new images based on existing ones or creates entirely new images. The creation of new images using generative AI techniques creates visually stunning and diverse artwork.
Techniques Used: DCGANs, VAEs, StyleGAN, PGGANs
Applications: Allows for the creation of unique and novel images Aids in artistic creativity and exploration Enhances machine learning models with data augmentation.
Text Generation
Text generation is a fascinating application of generative AI. Text generation aids in language-related tasks. It involves creating new text based on existing data or patterns. Here are key aspects of text generation:
1. Training data: Text generation models are trained on large datasets including books, articles, and social media posts. The more diverse and extensive the training data, the better the model’s ability to generate coherent and relevant text.
2. Language modeling: Text generation models use techniques like recurrent neural networks (RNNs) or transformers to understand language patterns. These models learn to predict the next word or sequence of words based on the input context.
3. Creative outputs: Text generation models can produce various outputs, from simple sentences to complex narratives. They are used for automated content generation, chatbots, language translation, and even generating lyrics or poetry.
4. Quality control: Ensuring the quality and coherence of the generated text is crucial. Evaluating the output against criteria like grammatical correctness, relevance, and fluency helps maintain the desired level of quality.
5. Application-specific fine-tuning: Text generation models can be fine-tuned for specific applications or domains. This involves training the model on a smaller dataset specific to the desired output, resulting in more targeted and accurate results.
Music Generation
Music generation allows for creativity and exploration in music composition. It is a fascinating sub-topic within generative AI. AI systems with this capability can create original compositions and melodies. There are three main methods for music generation:
- Traditional methods: These methods use existing musical notes and patterns to compose new music. They rely on predefined rules and structures to generate pieces.
- Neural networks: Neural networks analyze vast amounts of musical data to learn the patterns and structures of different genres or artists. They then generate new music based on these learned patterns.
- GANs: Generative Adversarial Networks (GANs) consist of two neural networks – a generator and a discriminator. The generator creates music, and the discriminator evaluates its quality, resulting in an iterative process that produces increasingly refined compositions.
Music generation through generative AI has immense potential for various applications. It can enhance creativity, inspire musicians, and assist in producing background scores for movies and games. It is important to note that AI-generated music lacks the emotional depth and contextual understanding that human musicians possess.
Pro-tip: When using AI-generated music, appreciate it as a tool for inspiration and creativity, rather than a complete replacement for human artistry.
Video Generation
Generative AI can also be used for video generation, virtual reality experiences, and data augmentation for machine learning. The possibilities are vast, limited only by imagination and technological advancements.
The Benefits and Limitations of Generative AI
Generative AI has revolutionized the fields of creativity, technology, and more. In this section, we’ll explore the exciting benefits and thought-provoking limitations associated with this cutting-edge technology.
Benefits
Generative AI offers a multitude of benefits. It increases efficiency by automating processes, thereby reducing manual labor and saving time and resources. It facilitates creative output by generating new and unique content, thereby aiding in the creation of original works.
Related: Generative AI In Workplaces
It enhances personalization by analyzing user preferences and behavior to provide customized experiences and recommendations.
Generative AI enables the exploration of possibilities by simulating scenarios and generating potential outcomes, thus allowing for the exploration of new ideas and possibilities.
It improves decision-making by analyzing data and generating insights, thereby assisting in data-driven decisions across industries. Importantly, generative AI tools are becoming more user-friendly and accessible, ensuring ease of accessibility and enabling individuals and businesses to harness AI benefits without specialized knowledge.
Generative AI also offers cost-effectiveness by automating tasks and reducing operational expenses. It increases productivity by generating creative and innovative outputs, thereby boosting overall productivity.
Limitations
Generative AI technology has its limitations, and it is crucial to be aware of these constraints. Here are some important limitations to consider:
1. Quality: It is important to note that the quality of generated outputs may vary. The content produced by generative AI models may not always meet the desired standards and may contain errors or inconsistencies. Therefore, it is essential to review and validate the generated output to ensure accuracy.
2. Dataset Dependence: To function effectively, generative AI models rely heavily on the quality and diversity of the datasets they are trained on. If the datasets used for training are insufficient or biased, it can lead to biased or incomplete generative outputs. To improve the performance of generative AI models, it is crucial to use comprehensive and representative datasets.
3. Lack of Creativity: Generative AI models learn from existing patterns and struggle to exhibit true creativity. They may have difficulty generating original and innovative content due to their heavy reliance on patterns observed in the training data.
4. Ethical Concerns: The use of generative AI raises ethical concerns, particularly regarding the potential misuse and manipulation of generated content. There is a risk of creating deceptive or misleading information, such as fake news or deepfake videos, using generative AI technology.
5. Computational Resources: Training and running generative AI models require substantial computational resources, including specialized hardware and large-scale computing infrastructure. Limited access to these resources can serve as a limiting factor for individuals or organizations interested in utilizing generative AI technology.
Despite these limitations, ongoing advancements in technology, research, and ethical frameworks can help address these challenges. It is crucial to recognize and understand these limitations to ensure responsible and informed use of generative AI technology.
Ethical Considerations in Generative AI
When it comes to generative AI, ethical considerations play a crucial role. In this section, we’ll dive into the fascinating realm of ethics and explore the thought-provoking sub-sections of fairness and bias, as well as misuse and manipulation. Prepare to uncover the moral challenges that arise when harnessing the power of generative AI, and discover the impact it has on our society and collective well-being. It’s time to delve into the ethics of generative AI and unravel its complexities.
Fairness and Bias
Fairness and bias are crucial considerations in the context of generative AI. It is imperative that AI algorithms acquire knowledge from unbiased and diverse data, as biased or insufficiently varied training data can lead to biased outcomes. In order to tackle this issue, it becomes necessary to establish ethical guidelines and standards throughout the development and implementation of generative AI systems. These guidelines should prioritize the minimization of biases within the data and the regular auditing of models to identify and rectify any biases present.
Transparency and accountability also hold immense significance, as they enable users to comprehend how the AI system operates and question any potential biases. It is essential to have diverse teams involved in the process, as they are better equipped to identify and address biases that homogeneous teams may inadvertently overlook. To achieve fairness, it is crucial to continuously monitor and test the models, utilizing techniques specifically designed to detect biases. The establishment of feedback loops and mechanisms that facilitate user input and the reporting of biases can further enhance the fairness and ethical nature of generative AI systems.
Misuse and Manipulation
Misuse and manipulation are significant concerns when it comes to generative AI. This technology has the power to produce deepfake videos, which are incredibly realistic yet entirely fabricated. These deceptive videos can be used to propagate false information, manipulate public opinion, and tarnish the reputation of individuals.
Generative AI can orchestrate the creation of counterfeit products or art, resulting in financial harm to consumers. To combat these issues, it is crucial to implement robust regulations and oversight.
The ethical development and deployment of generative AI should prioritize transparency and accountability. Conducting regular audits, enforcing strict guidelines, and imposing penalties for misuse can help deter unethical behavior and safeguard the well-being of individuals and businesses.
Future of Generative AI
The future of generative AI is buzzing with endless possibilities and exciting prospects. By delving into the advancements in technology and potential applications, we unravel the fascinating realm of this cutting-edge field.
Advancements in Technology
Advancements in technology have significantly contributed to the progress of generative AI. Enhanced hardware capabilities, efficient algorithms, and increased data availability are among the key advancements.
One particularly notable advancement is the utilization of deep learning algorithms, which have completely transformed the field of generative AI. These algorithms, like GANs and VAEs, are capable of generating outputs that are more realistic and of higher quality, spanning various media forms such as images, videos, and faces.
Another important advancement is the availability of larger datasets, which greatly enhance the learning process of the models and improve the accuracy of their generated outputs. With more easily accessible datasets, generative AI models are now able to produce content that is even more realistic and diverse.
The advancements in hardware capabilities, including powerful GPUs and the utilization of cloud computing, have significantly accelerated the training and inference processes. This results in faster and more efficient content generation for generative AI models.
Some Facts About What Is Generative AI? Everything You Need to Know:
- ✅ Generative AI refers to AI algorithms generating outputs, such as text, photos, videos, code, data, and 3D renderings, based on the data they are trained on. (Source: Our Team)
- ✅ Generative AI has gained popularity with programs like OpenAI’s ChatGPT and DALL-E, which can generate various types of content within seconds. (Source: Our Team)
- ✅ Generative AI has various applications in different industries, such as content generation, product design, simulations, gaming, and artificial synthesis of chemical compounds. (Source: Our Team)
- ✅ Generative AI is related to machine learning, as both use algorithms to learn from data. Generative AI focuses on creating new content, while machine learning focuses on classification, prediction, and clustering. (Source: Our Team)
- ✅ Generative AI enhances the customer experience in e-commerce by creating dynamic web content, analyzing customer data, and providing personalized recommendations and offers. (Source: Our Team)
Frequently Asked Questions
What is generative AI?
Generative AI refers to AI algorithms that can create new content, such as text, photos, videos, code, data, and 3D renderings, based on the data they are trained on. It utilizes machine learning algorithms to generate realistic and coherent outputs.
How does generative AI work?
Generative AI models work by starting with a prompt, which can be in the form of text, image, video, or any other input that the AI system can process. AI algorithms then generate new content in response to the prompt, combining different algorithms like natural language processing for text generation and visual processing for image generation.
These models are known as foundation models, which are large AI models that can multi-task and perform out-of-the-box tasks, including summarization, Q&A, classification, and more. Plus, with minimal training required, foundation models can be adapted for targeted use cases with very little example data from companies like IBM.
What are some popular generative AI tools?
Some popular generative AI tools include OpenAI’s GPT-4 (including ChatGPT), DALL-E (a tool for AI-generated art) TensorFlow, Pytorch, Keras, and AllenNLP. These tools provide pre-trained models and datasets, reducing computational power and data requirements. They also allow customization and fine-tuning for specific use cases.
What are the applications of generative AI?
Generative AI has various applications in different industries. It can be used for content generation, product design, simulations, gaming, and artificial synthesis of chemical compounds. It is also used in healthcare for generating new drugs, predictive healthcare, and improving mental health.
Other applications include workflow streamlining for creatives, engineers, researchers, scientists, fraud detection, customer service, predictive maintenance, enhancing customer experiences in retail and entertainment, and various other generative AI applications that have yet to be discovered, such as improving chat and search experiences for customers.
What are the benefits of generative AI?
Generative AI offers the ability to automate business functions, streamline operations, and create personalized experiences. It can automate content creation, reduce effort in responding to emails, improve technical queries, create realistic representations, summarize complex information, and simplify content creation.
What are the limitations and challenges of generative AI?
Generative AI has limitations and challenges such as identifying the source and bias of content, potential inaccuracies, disruption of existing business models, and the potential for misuse and abuse. It is important to ensure diverse and unbiased training data, address ethical considerations, and address concerns related to copyright and intellectual property rights to follow best practices for using generative AI.
- Understanding the Landscape of Cloud Vulnerability Management - March 25, 2024
- Quality Assurance Strategies for Startups: Ensuring Software Reliability - March 5, 2024
- Working of NLP to Improve Copywriting in AI Paraphrasing Tool - February 28, 2024