When it comes to the fascinating world of general AI, understanding the basics becomes crucial. In this article, we will dive into an introduction to general artificial intelligence (AGI) along with its definition and significance.
So, buckle up and get ready to explore the wonders of AGI and its approaches, which have the potential to revolutionize various industries and reshape the future of technology.
Are you curious to know what AGI truly entails and why it is such a game-changer? Let’s delve into the details and unravel the mysteries of general
Key Takeaway:
- General Artificial Intelligence (AGI) refers to the development of AI systems that possess human-like intelligence and can perform a wide range of tasks that would typically require human intelligence.
- Approaches to achieving AGI include the Symbolic Approach, which focuses on using rules and logic to simulate human reasoning, the Connectionist Approach, which utilizes artificial neural networks to mimic human brain functions, and the Hybrid Approach, which combines elements of both symbolic and connectionist approaches.
- The development of AGI faces key challenges, such as mastering human-like capabilities including sensory perception, motor skills, natural language understanding, and problem-solving abilities. These challenges require advancements in machine learning and deep learning techniques.
- Advancements in AGI continue to be made, with progress seen in symbolic approaches, breakthroughs in connectionist approaches, advancements in hybrid approaches, and the development of whole-organism architectures. These advancements contribute to the potential future implications and applications of AGI.
- The future of general artificial intelligence holds immense potential for various sectors and industries, including healthcare, finance, transportation, and more. AGI has the potential to revolutionize how tasks are automated and performed, leading to significant societal and economic impacts.
What Is General Artificial Intelligence?
General artificial intelligence (AGI) refers to the development of intelligent systems that possess human-like capabilities. AGI aims to create machines capable of perceiving, understanding, and problem-solving just as humans do.
This branch of AI goes beyond narrow task-specific applications and aims to achieve a broad range of cognitive abilities in machines.
AGI is characterized by its ability to perceive sensory inputs, develop motor skills, understand natural language, and solve complex problems. Mastering these human-like capabilities is essential for achieving AGI.
The development of general artificial intelligence (AGI) aims to create intelligent systems with human-like abilities such as sensory perception, motor skills, natural language understanding, and problem-solving capabilities.
AGI: The only thing scarier than an artificial intelligence that can outthink humans is an artificial intelligence that can outthink humans and has a dark sense of humor.
The Importance of Artificial General Intelligence (AGI)
The importance of AGI lies in its potential to revolutionize various industries and domains by enabling machines to perform tasks that currently require human intelligence.
This can lead to advancements in healthcare, robotics, autonomous vehicles, and other fields where computer systems can outperform humans in terms of speed and efficiency. AGI holds great promise for enhancing productivity and improving the quality of life for individuals across the globe.
The development of AGI involves several approaches, such as the symbolic approach, the connectionist approach, the hybrid approach, and whole-organism architecture.
Approaches to Achieving AGI: Whether it’s through symbols, connections, hybrids, or building an entire artificial organism, we’re trying everything but sacrificing souls (yet).
Approaches to Achieving AGI
When it comes to achieving general artificial intelligence (AGI), there are different approaches that researchers and experts have explored. We’ll uncover the strengths and limitations of each approach, shedding light on the symbolic approach, the connectionist approach, the hybrid approach, and the Whole-organism Architecture.
By exploring these different perspectives, we can gain a deeper understanding of the strategies employed in the pursuit of AGI.
The Symbolic Approach
This approach is based on the idea that intelligent behavior can be achieved by manipulating symbolic representations of information. In the Symbolic Approach, knowledge is represented using symbols, and relationships between symbols are defined through logical rules. These rules allow the system to perform tasks such as problem-solving, decision-making, and natural language understanding.
One advantage of the Symbolic Approach is its ability to handle complex reasoning tasks and provide explainable outputs. By using formal logic and well-defined rules, this approach can produce transparent results that can be easily understood by humans. Additionally, the Symbolic Approach allows for modularity in system design, making it easier to incorporate new knowledge or modify existing knowledge representations.
However, the Symbolic Approach also faces challenges in capturing real-world ambiguities and uncertainties. Its reliance on explicit symbol-based representation may limit its ability to handle complex sensory perception tasks or learn from large amounts of data efficiently. To overcome these limitations, researchers have been exploring hybrid approaches that combine symbolic reasoning with connectionist models or other techniques.
To further advance the development of General AI using the Symbolic Approach, researchers must continue refining symbolic representation methods and addressing key challenges such as handling uncertainty and incorporating more efficient learning mechanisms.
By doing so, we can unlock the potential of General AI in various domains such as healthcare, finance, education, and beyond. Don’t miss out on being part of this transformative journey towards achieving AGI through the Symbolic Approach.
The Connectionist Approach: Bringing brains and circuits together for a mind-blowing AI experience.
The Connectionist Approach
Significant progress has been made in the field of AI through the connectionist approach. Neural networks have shown remarkable capabilities in tasks such as image recognition, natural language processing, and speech synthesis. This approach has benefited from advancements in computing power and the availability of vast amounts of data for training purposes. Researchers are continually refining neural network architectures and developing more efficient learning algorithms to enhance their performance in specific tasks, leading to the development of powerful deep learning systems.
One key aspect of the connectionist approach is its ability to learn from data without explicit programming. Rather than being explicitly programmed with rules and logic, neural networks can analyze large datasets and identify complex patterns independently. This allows them to adapt and improve their performance over time through a process known as machine learning.
Overall, the connectionist approach provides valuable insights into achieving General Artificial Intelligence by emulating neural networks found in humans and animals. Through continuous advancements in this field, researchers aim to develop AI systems that can exhibit human-like cognitive abilities across various domains.
In a true story related to the connectionist approach, researchers at a leading AI laboratory used deep learning models based on neural networks to create an AI system capable of beating world chess champions.
This groundbreaking achievement showcased the power and potential of the connectionist approach in developing highly capable AI systems. By analyzing vast amounts of chess game data, the system was able to acquire strategic knowledge and make intelligent moves during gameplay, ultimately outperforming even seasoned human players in competitive matches. This success story highlights the effectiveness and potential of the connectionist approach in achieving General Artificial Intelligence.
The Hybrid Approach: When it comes to achieving AGI, these researchers aren’t playing favorites – they’re mixing and matching like a mad scientist with a budget.
The Hybrid Approach
The Semantic NLP Variation of ‘The Hybrid Approach‘ can be explained as the combination and integration of different AI techniques, such as symbolic and connectionist approaches, to achieve General Artificial Intelligence (AGI).
The Hybrid Approach can be better understood through a table that highlights its key features:
Features | Symbolic Approach | Connectionist Approach |
---|---|---|
Strengths | Logic-based reasoning, rule-based systems | Pattern recognition, parallel processing |
Weaknesses | Lack of flexibility, inability to handle large amounts of data | Difficulty in interpreting results, lack of explainability |
Usage | Expert systems, rule engines | Deep learning, neural networks |
In addition to the features mentioned above, The Hybrid Approach also combines the strengths and compensates for weaknesses of both symbolic and connectionist techniques. This includes using symbolic approaches for logical reasoning and rule-based decision-making while harnessing connectionist techniques for pattern recognition and handling large datasets.
One example highlighting the effectiveness of The Hybrid Approach is the development of self-driving cars. By integrating symbolic reasoning for object recognition and decision-making with connectionist methods for image processing and real-time responsiveness, researchers have been able to create autonomous vehicles capable of navigating complex road scenarios.
Overall, The Hybrid Approach represents a promising direction in the pursuit of AGI as it combines the best aspects of different AI techniques to address their respective limitations while leveraging their strengths in tandem.
Hold on to your circuit boards, because we’re about to explore the mind-boggling concept of Whole-organism Architecture in the world of General Artificial Intelligence!
Whole-organism Architecture
The whole-organism architecture refers to a novel approach to achieving General Artificial Intelligence (AGI). This approach emphasizes the development of AI systems that mimic the structure and functionalities of living organisms.
Unlike other approaches, it focuses on creating AI systems that encompass all aspects of an organism, including sensory perception, motor skills, natural language understanding, and problem-solving abilities. In this context, the whole-organism architecture aims to replicate the complexity and adaptability observed in biological organisms.
By designing AI systems with a holistic perspective, researchers hope to overcome the limitations of traditional approaches and enable AGI to possess human-like capabilities. A key characteristic of the whole-organism architecture is its integration of multiple components and subsystems that work together in harmony.
For example, sensory perception modules interact with motor skills modules to enable intelligent decision-making and action. Furthermore, this approach acknowledges the importance of considering both cognitive and physical aspects when developing AI systems.
By exploring the potential of the whole-organism architecture, researchers anticipate significant advancements in AGI capabilities. This approach has the potential to advance areas such as robotics, autonomous vehicles, healthcare diagnostics, and even human-computer interfaces. Embracing the concept of whole-organism architecture is crucial in pushing the boundaries of AGI research.
The integration of cognitive abilities with physical embodiment can lead to breakthroughs not only in AI technology but also in our understanding of intelligence itself. Missing out on exploring this promising avenue could hinder progress toward achieving true General Artificial Intelligence and artificial superintelligence. Mastering human-like capabilities in a robot is no easy task, but once it starts beating us at rock-paper-scissors, we’ll know we’re in trouble in the real world.
Key Challenges in Reaching the General AI Stage
When it comes to advancing artificial intelligence (AI) towards the goal of general AI, one of the key challenges lies in mastering human-like capabilities. This is where the true complexity and demands of developing AI systems come into play.
In this section, we will delve into the specific hurdles that researchers and developers face when trying to replicate human cognitive abilities in machines. The exploration of these challenges will shed light on the intricacies involved in achieving the general AI stage.
According to the reference data, scientists at OpenAI have been working tirelessly on these challenges, aiming to push the boundaries of AI capabilities.
Issues In Mastering Human-like Capabilities
Human-like capabilities refer to the ability of artificial general intelligence (AGI) systems to replicate and surpass the cognitive abilities and skills exhibited by humans. These include sensory perception, motor skills, natural language understanding, and problem-solving abilities.
The aim is for AGI systems to possess these capabilities at a level that matches or exceeds human performance. To achieve this, researchers are exploring various approaches such as symbolic, connectionist, hybrid, and whole-organism architectures.
To master human-like capabilities, AGI systems need to advance in sensory perception by accurately perceiving and interpreting their environment through vision, hearing, touch, and other senses.
They should also develop sophisticated motor skills that enable them to manipulate objects and perform complex tasks with precision. Additionally, natural language understanding is crucial for AGI systems to comprehend and generate human-language text in a manner that resembles genuine human understanding.
Lastly, the development of problem-solving abilities is essential for AGI systems to effectively analyze complex problems, devise strategies and solutions, and adapt to changing circumstances.
To enhance these capabilities further:
- Provide extensive training data in diverse environments for sensory perception.
- Implement reinforcement learning algorithms for refining motor skills based on feedback.
- Utilize deep learning techniques combined with contextual understanding for achieving advanced natural language processing.
- Incorporate machine learning algorithms capable of probabilistic reasoning for more sophisticated problem-solving.
By continuously improving these aspects of AGI systems’ capabilities, we can strive towards creating truly human-like artificial intelligence that surpasses current limitations in cognitive functioning. The future of AI is looking bright, or should I say, sensor-y!
Get ready for machines that can see, hear, smell, taste, and touch—just don’t ask them for fashion advice.
Sensory Perception
Developing advanced sensory perception capabilities is crucial for AGI, as it enables the AI system to perceive and process data from different sources, such as visual images, audio signals, and tactile feedback. By analyzing this information, an AI system can make informed decisions and respond effectively to its surroundings.
To enhance sensory perception in AGI, researchers are exploring various techniques such as computer vision algorithms for visual perception, speech recognition technologies for auditory perception, and haptic interfaces for tactile perception. These advancements enable AI systems to process and interpret sensory data more accurately, mimicking human-like perception.
Improving sensory perception in AGI has wide-ranging implications across various fields such as autonomous vehicles, robotics, healthcare, and virtual reality. For example, enhanced visual perception can be utilized in self-driving cars to identify objects on the road accurately. Similarly, precise auditory perception can help in developing voice-controlled personal assistants that understand natural language commands more effectively.
To further enhance sensory perception in AGI systems, researchers should focus on improving the accuracy and reliability of sensing technologies. Additionally, integrating multiple modalities of sensory input can provide a richer understanding of the environment. By combining visual, auditory, and tactile information seamlessly, AGI systems can achieve more comprehensive sensory perception capabilities.
We can enable AGI systems with enhanced perceptual abilities that closely resemble human sensory experiences.
AGI’s quest for motor skills: it’s like teaching a robot to do the floss… without any rhythm.
Motor Skills
Motor skills refer to the ability of an artificial intelligence system to control and manipulate physical objects or perform physical tasks with precision and coordination. It involves the AI’s capability to engage in activities that require fine motor movements, such as grasping, reaching, and manipulating objects.
In the context of AGI development, mastering motor skills is a crucial aspect. It enables an AI system to interact with its environment effectively and carry out various tasks in a human-like manner. This includes tasks like object manipulation, tool usage, locomotion, and even more complex actions like playing musical instruments or sports.
While significant advancements have been made in developing AI systems with motor skills capabilities, there are still challenges that need to be addressed. These include improving dexterity and accuracy of movements, enhancing adaptability to different environmental conditions, and refining the ability to learn new motor skills through observation and practice.
Developing AI systems with advanced motor skills has wide-ranging implications across various industries and sectors. For example, it can revolutionize manufacturing processes by enabling robots to perform intricate assembly tasks with higher efficiency and precision.
It can also enhance healthcare by assisting surgeons in performing delicate surgical procedures or aiding individuals with physical disabilities in regaining independence through the use of assistive technologies.
Given the transformative potential of AGI with advanced motor skills, it is essential for researchers and developers to continue pushing boundaries in the ai field. By investing in research and development efforts focused on improving the motor skills capabilities of AI systems, we can unlock new possibilities for automation, productivity enhancement, and overall societal progress.
Additionally, the concept of AGI with advanced motor skills is a common topic in science fiction and futures studies, showcasing the potential impact and implications of this technology.
Don’t miss out on the opportunity to shape the future of technology by advancing AGI’s motor skills capabilities.
Understanding what AI says is like deciphering a hangry toddler’s tantrums.
Natural Language Understanding
The field of Natural Language Understanding (NLU) focuses on the ability of AI systems to comprehend and interpret human language. NLU enables machines to understand and derive meaning from text or speech, allowing them to interact with humans more naturally and intuitively.
In the context of General Artificial Intelligence (AGI), achieving advanced levels of natural language understanding is crucial. It involves enabling AI systems to not only parse and recognize words but also grasp the nuances, context, and intention behind human language. This includes understanding syntactic structures, semantic relationships, linguistic patterns, idiomatic expressions, and even cultural references and common sense, as well as the ability to solve new problems that they didn’t know about at the time of their creation.
Developing robust natural language understanding capabilities presents significant challenges. AI systems need to be capable of accurately interpreting diverse forms of human communication across different languages and dialects.
They must also possess reasoning abilities to analyze complex sentences and extract relevant information effectively. Furthermore, they should be able to adapt their understanding based on changes in context or user preferences.
Historically, significant progress has been made in improving natural language understanding through various approaches, such as machine learning techniques combined with linguistic models and knowledge graphs.
These advancements have paved the way for applications including voice assistants, chatbots, sentiment analysis, document summarization, translation services, and more.
Problem-solving Abilities
Problem-solving abilities refer to the cognitive skills and processes involved in identifying, analyzing, and solving problems. These abilities enable an artificial intelligence system to understand complex issues, generate possible solutions, and evaluate their potential effectiveness.
- Recognize the Problem: The first step in problem-solving is to identify and define the problem. AI systems need to be able to understand and interpret the problem statement accurately.
- Analyze the Problem: Once the problem is recognized, AI systems need to analyze it by gathering relevant information and breaking it down into smaller components or sub-problems. This analysis helps in better understanding the problem’s nature and constraints.
- Generate Solutions: After analyzing the problem, AI systems must generate possible solutions or strategies for solving it. This requires creativity, adaptability, and considering various approaches.
- Evaluate Solutions: Finally, AI systems need to evaluate each generated solution based on predetermined criteria or objectives. This evaluation involves assessing the feasibility, efficiency, and potential consequences of each solution before selecting the most appropriate one.
For artificial intelligence systems to have effective problem-solving abilities, they must exhibit a high level of adaptability, reasoning capability, flexibility, computational power, and access to vast amounts of data. These features allow them to navigate complex problems across various domains efficiently.
Progress in developing problem-solving abilities has been observed through advancements in symbolic approaches like logic-based reasoning systems and knowledge representation techniques.
Additionally, breakthroughs in connectionist approaches using neural networks have also contributed significantly to enhancing problem-solving capabilities. Hybrid approaches combining both symbolic and connectionist methods have shown promising results as well.
To further advance general artificial intelligence’s problem-solving abilities successfully, researchers are exploring novel techniques such as whole-organism architectures that mimic human-like cognitive processes.
By continually improving its problem-solving abilities across different domains and expanding its knowledge base through machine learning algorithms, artificial general intelligence can revolutionize sectors like healthcare, finance, transportation, and manufacturing.
In a true story reported by researchers, an AI system with exceptional problem-solving abilities was able to diagnose a rare medical condition accurately. By analyzing vast amounts of patient data and medical research papers, the AI system identified patterns that eluded human doctors. This breakthrough resulted in early interventions and improved patient outcomes.
In a true story reported by researchers, an AI system with exceptional problem-solving abilities, also known as strong AI, was able to diagnose a rare medical condition accurately. By analyzing vast amounts of patient data and medical research papers, the strong AI system identified patterns that eluded human doctors. This breakthrough resulted in early interventions and improved patient outcomes. The problem-solving abilities of this strong AI system showcase the potential of general AI and its potential to revolutionize industries and improve our daily lives, similar to the way Amazon’s recommendation system has transformed the online shopping experience.
AI’s problem-solving abilities may one day surpass us, but for now, it’s mostly just really good at finding the answer to ‘What’s the weather like?
Latest Trends in General Artificial Intelligence
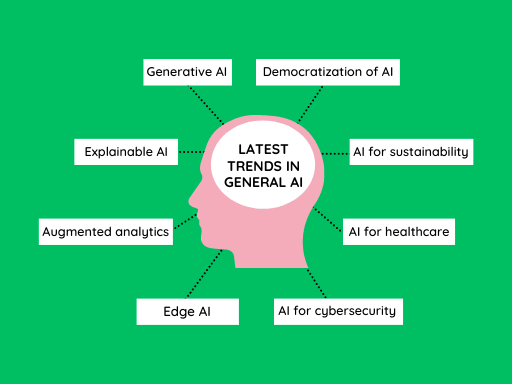
In the world of general artificial intelligence (AI), advancements continually shape the landscape of this field. Let’s explore the latest trends driving progress in AI.
- Democratization of AI: AI is becoming more accessible to businesses and individuals of all sizes, thanks to the development of low-code and no-code AI platforms. This is enabling more people to use AI to solve problems and improve their businesses.
- Generative AI: Generative AI is a type of AI that can create new content, such as images, text, and music. This is being used in a variety of industries, including marketing, healthcare, and entertainment.
- Explainable AI: Explainable AI is a type of AI that can explain its decisions. This is important for ensuring that AI is used in a responsible and ethical way.
- Augmented analytics: Augmented analytics is a type of analytics that combines AI with traditional analytics techniques. This makes it easier for businesses to find insights from their data.
- Edge AI: Edge AI is a type of AI that is deployed on devices at the edge of the network. This makes it possible for AI to be used in real-time and in remote locations.
- AI for cybersecurity: AI is being used to improve cybersecurity by detecting and preventing cyberattacks. This is becoming increasingly important as cyberattacks become more sophisticated.
- AI for healthcare: AI is being used to improve healthcare by diagnosing diseases, recommending treatments, and providing personalized care. This is having a significant impact on the quality and efficiency of healthcare.
- AI for sustainability: AI is being used to improve sustainability by reducing energy consumption, optimizing transportation, and managing waste. This is helping to address some of the most pressing challenges facing our planet.
These are just a few of the latest trends in general AI. As AI continues to develop, we can expect to see even more exciting and groundbreaking applications in the years to come.
General Artificial Intelligence Advancements: Watch out for symbolically clever, hybrid-connected, and whole-organismically impressive progress in the AI realm.
Advancements General AI
We’ll look into the breakthroughs in connectionist approaches, advancements in symbolic approaches, progress in hybrid approaches, and the development of whole-organism architectures. By understanding these advancements, we can gain insights into the future implications and applications of AGI (artificial general intelligence).
It is an exciting time as AI pushes boundaries and opens up new horizons for technology and human interaction.
Advancements in Symbolic Approaches
Symbolic approaches in the field of artificial intelligence have witnessed remarkable advancements. These innovations have contributed to the development and progress of general artificial intelligence (AGI). Through the use of symbols and rules, symbolic approaches aim to emulate human-like intelligence and problem-solving abilities.
This approach has seen significant breakthroughs in various areas such as natural language understanding, pattern recognition, and logical reasoning. Researchers continue to refine symbolic algorithms and techniques, pushing the boundaries of AGI capabilities.
The advancements in symbolic approaches have paved the way for more sophisticated AI applications.
By leveraging symbolic representations, AI systems can better understand complex concepts, interpret vast amounts of data, and make intelligent decisions. These developments have enabled AI systems to excel in tasks that require logical reasoning, such as medical diagnosis, image recognition, and language translation.
However, further research is still needed to overcome certain challenges associated with symbolic approaches. One key challenge lies in the scalability and efficiency of these methods when dealing with large-scale datasets or complex real-world scenarios.
To address this limitation, researchers are exploring hybrid approaches that combine both symbolic and connectionist techniques to leverage their respective strengths.
Buckle up, because these connectionist approaches are about to blow your mind and reshape the future of artificial intelligence.
Breakthroughs in Connectionist Approaches
The recent progress in connectionist approaches has resulted in significant breakthroughs in the development of general artificial intelligence (AGI). Researchers have made groundbreaking advancements in the implementation of neural networks and deep learning algorithms, enabling machines to learn and process information in a manner that simulates human cognitive abilities.
These breakthroughs have revolutionized fields such as image recognition, natural language processing, and speech synthesis, bringing us closer to achieving AGI.
These breakthroughs in Connectionist Approaches have propelled the field of AI forward by developing more sophisticated models and architectures. One notable advancement is the creation of deep neural networks with multiple layers, allowing for more complex and accurate predictions.
Additionally, researchers have made progress in optimizing training algorithms, improving the efficiency and speed of learning processes. The application of Connectionist Approaches has also opened doors to advancements in areas such as robotics, autonomous vehicles, and healthcare.
Moreover, breakthroughs in Connectionist Approaches have paved the way for important applications of AGI across various industries. For example, in healthcare, AI systems utilizing connectionist approaches can analyze vast medical datasets to identify patterns and make accurate diagnoses.
In finance, these breakthroughs enable the development of advanced trading algorithms that can predict market trends with greater accuracy. Furthermore, advancements in natural language processing have led to the development of virtual assistants capable of understanding and responding to human speech.
Significant progress has been made due to breakthroughs in Connectionist Approaches within a relatively short period. This exponential growth is attributed to advances such as improved hardware capabilities, increased availability of data for training purposes, and continuous research efforts by experts worldwide.
The successful integration of connectionist approaches into AI systems has brought us closer to realizing the potential of AGI and opened up new possibilities for how we interact with intelligent machines.
Hybrid approaches in AGI development: bringing together the best of both symbol manipulation and neural networks, like a peanut butter and jelly sandwich of artificial intelligence.
Progress in Hybrid Approaches
Text: Hybrid Approaches: Advancements and Integration of Symbolic and Connectionist Methods
The progress in hybrid approaches, which involve combining symbolic and connectionist methods, has been notable in the field of general artificial intelligence (AGI).
This integration aims to harness the strengths of both approaches to overcome their individual limitations.
Symbolic Approach | Connectionist Approach | Hybrid Approach |
– Use of logical rules and representations | – Utilization of artificial neural networks | – Combination of symbolic reasoning and connectionist learning |
– Emphasis on explicit reasoning | – Focus on learning through data-driven methods | – Enhanced performance in handling complex tasks |
– Limited ability to handle uncertainty and complex patterns | – Challenges with explainability and interpretability | – Overcoming limitations of purely symbolic or connectionist approaches |
Within the realm of hybrid approaches, researchers have made significant strides by developing innovative architectures that integrate symbolic reasoning with deep learning techniques. These advancements have resulted in improved problem-solving capabilities, natural language understanding, and decision-making abilities.
Furthermore, the progress in hybrid approaches has brought about exciting opportunities for various applications. Industries such as healthcare, finance, robotics, and autonomous systems can benefit from AGI systems that combine the best features of both symbolic and connectionist methods.
From neurons to networks, AGI is evolving with a touch of Frankenstein’s creativity.
Development of Whole-organism Architectures
Whole-organism architectures refer to the advancement and implementation of AI systems that mimic the holistic structure and functioning of living organisms. This approach aims to create AI systems that can integrate and simulate various cognitive abilities at once, enabling a more comprehensive and human-like intelligence.
Here is a 3-step guide to understanding the development of whole-organism architectures:
- Integration of Cognitive Abilities: The development of whole-organism architectures involves integrating multiple cognitive abilities, such as sensory perception, motor skills, natural language understanding, and problem-solving abilities. By combining these capabilities into a cohesive system, researchers aim to achieve a more generalized form of artificial intelligence.
- Mimicking Biological Processes: Whole-organism architectures draw inspiration from biological processes in order to design AI systems that can replicate the complex interactions and feedback loops found in living organisms. This includes modeling neural networks after the structure of the brain or utilizing evolutionary algorithms to optimize AI performance.
- Real-world Application: The ultimate goal of developing whole-organism architectures is to create AI systems that can operate in real-world environments with human-like adaptability and intelligence. Such systems could revolutionize industries ranging from healthcare and robotics to finance and transportation.
It is essential to explore the development of whole-organism architectures as it represents a significant step towards achieving general artificial intelligence. By simulating the integration of cognitive abilities found in living organisms, we can unlock new possibilities for AI systems and their applications. Don’t miss out on being part of this groundbreaking research and innovation! Join us on our quest for creating truly intelligent machines that can navigate the complexities of our world with finesse and ingenuity.
AGI’s future implications could range from revolutionizing industries to taking over the world, but let’s focus on the positive for now.
Future Implications and Applications of AGI
General Artificial Intelligence (AGI) has immense potential in shaping the future and revolutionizing various industries. As AGI continues to advance, its future implications and applications become increasingly profound.
The development of AGI can lead to groundbreaking advancements in fields such as healthcare, finance, transportation, and manufacturing. Moreover, AGI has the potential to transform the way we live and work, offering innovative solutions to complex problems and enhancing human capabilities.
One key challenge in achieving general AI is ensuring ethical and responsible use. As AI becomes increasingly sophisticated, it is crucial to establish guidelines and regulations to prevent misuse or potential harm.
Another significant aspect to consider is the integration of general AI into different industries. As AI technology evolves, businesses across sectors can leverage its potential to streamline processes, enhance productivity, and drive innovation.
To ensure the successful integration of general AI, collaboration between different stakeholders is paramount. Academia, industry experts, policymakers, and regulatory bodies must work together to address challenges, develop ethical frameworks, and foster responsible AI development and deployment.
Conclusion: The Future of General Artificial Intelligence
The future of general artificial intelligence (AI) holds immense potential and excitement. As AI continues to advance, it is expected to revolutionize various industries and aspects of our lives. However, some significant challenges and trends shape this journey.
In the future, we can anticipate the further development and refinement of general AI capabilities. This includes advancements in natural language processing, deep learning, and neural networks, enabling machines to understand and process information more effectively.
Some Facts About What Is General Artificial Intelligence (AI)?:
- ✅ Artificial general intelligence (AGI) refers to machines that mimic human tasks and solve complex problems. (Source: Team Research)
- ✅ AGI is based on the theory of mind AI framework, which trains machines to learn human behavior and understand consciousness. (Source: Team Research)
- ✅ The core approaches to AGI include the symbolic approach, connectionist approach, hybrid approach, and whole-organism architecture. (Source: Team Research)
- ✅ Sensory perception, motor skills, natural language understanding, and problem-solving are some of the human-like capabilities that AGI needs to master. (Source: Team Research)
- ✅ Achieving AGI has challenges related to simulation time, learning lower-level tasks, and mastering physical interactions. (Source: Team Research)
FAQs about What Is General AI?
What are the challenges in achieving General Artificial Intelligence?
There are several challenges in achieving General Artificial Intelligence, such as mastering human-like capabilities including sensory perception, motor skills, natural language understanding, and problem-solving. Additionally, the development of comprehensive knowledge, cognitive computing, and handling uncertain situations pose significant challenges.
What are the trends in advancements in General Artificial Intelligence?
Some of the key trends in advancements of General Artificial Intelligence include the use of symbolic approaches, connectionist approaches like neural networks, hybrid approaches that combine symbolic and sub-symbolic systems, and the exploration of whole-organism architectures. These trends aim to enhance the capabilities and performance of machine intelligence.
What is the theory of mind AI framework?
The theory of mind AI framework deals with training machines to learn human behavior and understand the fundamental aspects of consciousness. It forms the foundation for Artificial General Intelligence (AGI) by allowing machines to plan, learn cognitive abilities, make judgments, handle uncertain situations, and integrate prior knowledge in decision-making or improve accuracy.
This framework was developed by the renowned computer scientist and mathematician, Alan Turing, through his famous Turing Test.
How can General Artificial Intelligence perform innovative, imaginative, and creative tasks?
General Artificial Intelligence (AGI) has the ability to perform innovative, imaginative, and creative tasks by emulating the human mind and behavior. By comprehending, learning, and simulating human intelligence, AGI machines can approach problem-solving in a way that resembles human creativity and adaptability.
What are the approaches to achieving General Artificial Intelligence (AGI)?
There are several approaches to achieving General Artificial Intelligence (AGI). These include the symbolic approach, which utilizes logic networks and symbols to develop a comprehensive knowledge base; the connectionist approach, which uses architectures resembling the human brain, such as neural nets; the hybrid approach, which combines symbolic and sub-symbolic systems; and the concept of whole-organism architecture, involving physical bodies and learning from physical interactions.
- Understanding the Landscape of Cloud Vulnerability Management - March 25, 2024
- Quality Assurance Strategies for Startups: Ensuring Software Reliability - March 5, 2024
- Working of NLP to Improve Copywriting in AI Paraphrasing Tool - February 28, 2024